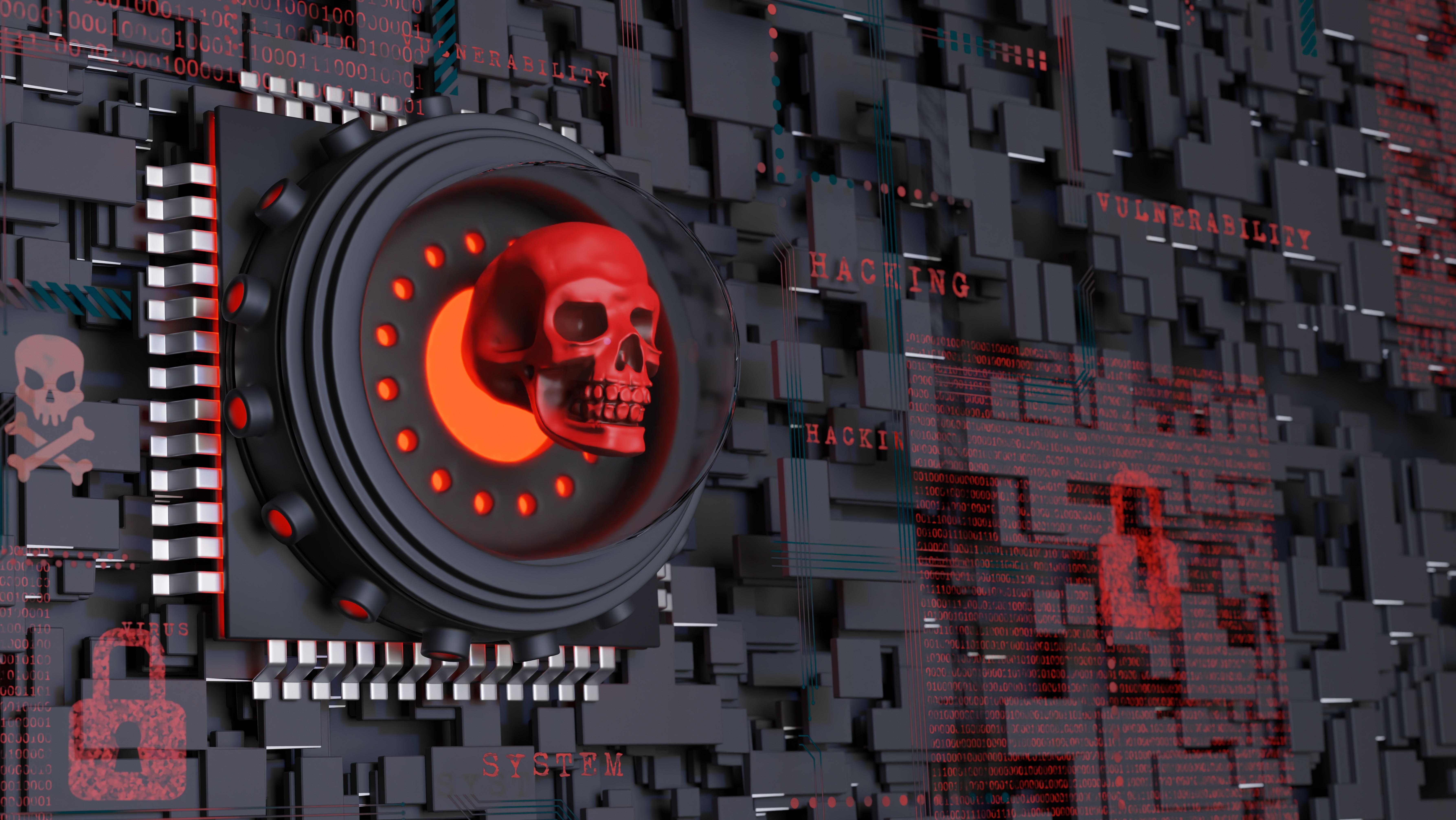
Artificial intelligence has become an integral part of modern business and cybersecurity, powering everything from automated decision-making to threat detection. However, as AI adoption accelerates, so too do the risks associated with its deployment.
AI Threats. Key Findings from 2025
According to industry surveys:
- 74% of organizations reported an AI-related breach in 2024, up from 67% the previous year.
- 45% of AI security incidents originated from malware embedded in public AI model repositories.
- 89% of IT leaders confirmed that AI models in production are critical to their business operations.
These statistics underscore the urgency of addressing AI security risks, particularly as adversaries develop more advanced attack techniques, such as data poisoning, adversarial ML attacks, and AI-powered phishing campaigns.
Why Do Attackers Use These Techniques?
Cybercriminals exploit AI vulnerabilities to gain financial, strategic, or disruptive advantages. Data poisoning allows them to corrupt AI models, leading to flawed decision-making in fraud detection, automated threat analysis, or medical diagnostics. By manipulating training data, attackers can degrade model performance or introduce hidden backdoors, ensuring long-term exploitation without immediate detection. Adversarial machine learning attacks take this a step further by crafting imperceptible manipulations that force AI to misclassify inputs, bypassing security controls or altering automated decision-making systems.
Meanwhile, AI-powered phishing campaigns leverage machine learning to create hyper-personalized and convincing messages, making social engineering attacks more effective. Attackers use AI to analyze large datasets, mimicking communication styles and automating large-scale phishing efforts with minimal effort. These techniques enable them to steal credentials, distribute malware, or manipulate users into revealing sensitive information, significantly increasing the success rate of cyberattacks.
Emerging AI Security Challenges
Supply Chain Vulnerabilities
AI systems rely on vast datasets and third-party models, many of which are sourced from public repositories like Hugging Face and Azure. Attackers increasingly embed malicious code into these models, compromising enterprises that fail to conduct proper vetting and validation. A notable example occurred in 2024 when researchers discovered multiple AI models in open repositories containing hidden malware, allowing attackers to hijack computing resources and exfiltrate sensitive data once deployed in enterprise environments.
Another major risk is dependency hijacking, where cybercriminals inject malicious components into AI frameworks or libraries that developers unknowingly incorporate into their projects. This tactic has been observed in recent attacks targeting AI-driven automation tools, where poisoned dependencies enabled adversaries to gain persistent access to corporate networks.
Adversarial Machine Learning Attacks
Cybercriminals are refining methods such as adversarial perturbations, model evasion, and data poisoning to manipulate AI outputs. These tactics can be used to deceive AI-driven fraud detection systems, bypass authentication mechanisms, or spread disinformation. For instance, attackers have successfully used adversarial patches—small, seemingly benign modifications to images—that trick AI-powered surveillance cameras into misclassifying objects or failing to detect threats.
A well-documented case in 2024 involved a research team demonstrating how slight modifications to an image of a stop sign caused self-driving car AI systems to misinterpret it as a speed limit sign, posing severe safety risks. Similarly, data poisoning has been leveraged by threat actors to manipulate AI-powered recruitment tools, causing biased hiring decisions by introducing skewed data samples.
Governance and Compliance Gaps
Despite the growing adoption of AI, regulatory frameworks remain inconsistent across industries and regions. A lack of clear security standards leaves organizations exposed to legal, ethical, and operational risks. Many enterprises struggle with AI governance, particularly regarding transparency in AI decision-making and accountability for AI-generated outputs.
One high-profile example is the ongoing debate surrounding AI-generated deepfake evidence in legal proceedings. The absence of universally accepted authentication mechanisms has led to concerns about the admissibility of AI-generated content in courts. Furthermore, industries such as finance and healthcare face increasing pressure to comply with evolving AI regulations, yet many organizations lack the necessary tools to enforce compliance effectively.
Deepfake and Social Engineering Exploits
AI-generated deepfakes and synthetic media have emerged as powerful tools for misinformation, fraud, and identity theft. Attackers are using generative AI to craft highly realistic phishing emails, clone voices, and impersonate executives in business email compromise (BEC) schemes. In one case from 2024, fraudsters used an AI-generated voice clone of a company’s CFO to authorize fraudulent wire transfers totaling millions of dollars.
Similarly, AI-generated fake news and political misinformation campaigns have been on the rise. During the 2024 election cycle, threat actors distributed AI-generated videos impersonating political figures, misleading voters, and influencing public opinion. These deepfake campaigns, often amplified through social media bots, illustrate how AI-driven disinformation poses a growing threat to global security and democracy.
AI Defenses. Best Practices for 2025
With 96% of organizations increasing their AI security budgets, industry leaders are prioritizing several key defensive measures:
Integrating AI Security into Development Lifecycles. Organizations must adopt security-by-design principles, embedding threat modeling, adversarial testing, and model verification into AI development pipelines.
Implementing AI Red Teaming Exercises. Simulated attacks help identify vulnerabilities before real adversaries exploit them. AI red teaming is becoming a standard practice in enterprises adopting AI at scale.
Adopting Zero Trust Architectures for AI Workloads. Strict access controls, continuous monitoring, and encryption at rest and in transit are essential to protect AI models and training data.
Enhancing AI Incident Response Plans. Security teams must develop specialized response playbooks for AI-related incidents, ensuring rapid containment and forensic analysis.
Regulatory Compliance and Ethical AI Governance. As new legislation emerges, companies must align their AI security strategies with evolving regulatory requirements, including transparency mandates and bias mitigation techniques.
AI Security Predictions
As we move beyond 2025, AI security will become an even more critical aspect of digital infrastructure. The increasing sophistication of AI-driven threats necessitates proactive strategies to mitigate risks and enhance security frameworks. Below are some of the key developments expected in AI security:
Increased AI-Specific Regulations
Governments and industry regulators will implement stricter compliance requirements to address AI-related risks. Expect new mandates on transparency, data privacy, and AI-generated content attribution, ensuring that organizations disclose how AI models make decisions, what data they use, and whether their outputs are reliable. The European Union’s AI Act and similar regulations in the United States, China, and other global markets will shape how businesses handle AI governance. Organizations will need to adopt AI ethics policies, model auditing practices, and bias mitigation techniques to align with these evolving standards.
Greater Use of AI for Defensive Cybersecurity
AI is not only a security risk but also a valuable tool for cyber defense. AI-driven security systems will become more autonomous, adaptive, and efficient in detecting cyber threats in real-time. Threat detection platforms will increasingly leverage machine learning for behavioral analysis, allowing organizations to identify anomalies and potential breaches faster. Additionally, AI-powered automated incident response will reduce reaction times, allowing security teams to mitigate threats before they escalate. The use of AI-driven deception technology, such as honeypots powered by machine learning, will also grow to mislead and track attackers.
Evolving Threats in AI Supply Chains
Attackers are shifting their focus to AI supply chains, targeting model repositories, APIs, and edge deployments. The growing reliance on open-source AI models makes organizations vulnerable to dependency hijacking, poisoned datasets, and backdoored machine learning models. Expect new verification and validation processes, such as cryptographic model signing and provenance tracking, to counteract these risks. Companies will need to implement continuous monitoring of AI pipelines to detect unauthorized modifications and hidden threats before deployment.
AI-Augmented Social Engineering Attacks
As deepfake technology improves, AI-generated phishing campaigns and impersonation scams will become more difficult to detect. Attackers will use AI to analyze speech patterns, writing styles, and digital footprints to create highly personalized attacks. For instance, voice cloning attacks will allow cybercriminals to mimic executives in real-time, authorizing fraudulent transactions or leaking sensitive information. To counteract these threats, organizations must adopt multi-factor authentication, behavioral biometrics, and AI-powered fraud detection systems that can identify manipulated media and anomalous interactions.
Final Thoughts
AI is transforming industries at an unprecedented pace, but without robust security measures, its benefits can be undermined by emerging threats. Organizations must invest in AI security frameworks, regulatory compliance, and adversarial testing to safeguard their AI infrastructure. As threats evolve, collaboration between governments, private sector leaders, and cybersecurity experts will be essential in building resilient AI ecosystems. The future of AI security depends on continuous adaptation, innovation, and vigilance against new attack vectors.
The future of AI security depends on proactive defense, regulatory alignment, and continuous adaptation to evolving threats.
Don’t just keep up with trends — be prepared for them!
Test our platform: https://a42.tech/